Data Lakes
A data lake can be defined as a centralized repository that stores large volumes of structured, semi-structured, and unstructured data. It is designed to accommodate data in its raw format, without the need for predefined schema or data transformations. The concept of a data lake originated from the need to store and analyze big data, which often comes from a wide variety of sources and exhibits high velocity and volume.
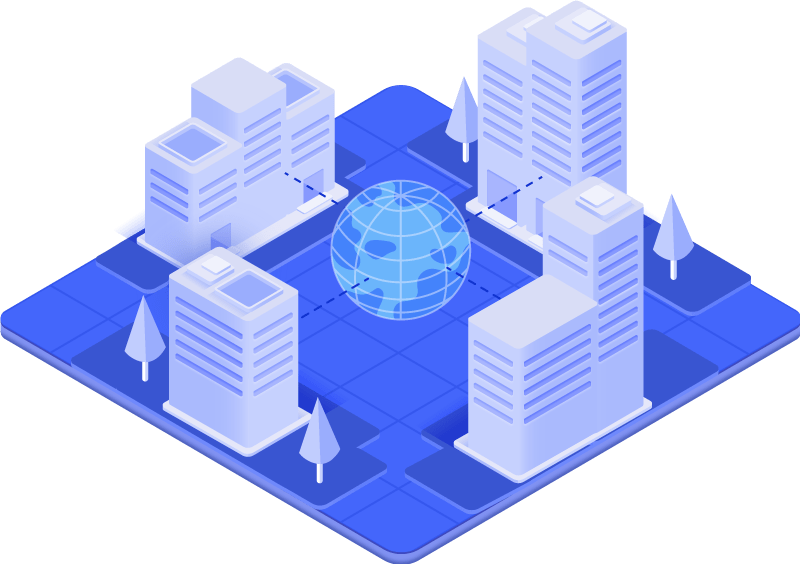
Implementing a data lake offers several significant benefits to organizations:
a) Data Discovery and Exploration: Data lakes facilitate the discovery of new insights by providing a comprehensive and unified view of data from different sources. Users can explore data without predefined schemas, enabling agile and ad-hoc analysis.
b) Data Integration and Consolidation: Data lakes enable the consolidation of data from disparate sources, breaking down data silos and providing a holistic view of an organization’s data assets.
c) Scalable Storage and Processing: Data lakes leverage cloud-based infrastructure, ensuring scalability and elasticity to handle growing data volumes and computational requirements.
d) Cost Optimization: Data lakes allow organizations to optimize costs by leveraging cloud storage and computing resources, paying only for what they use.
e) Advanced Analytics and Machine Learning: Data lakes provide a rich and diverse data set for advanced analytics and machine learning algorithms, enabling organizations to derive valuable insights and make data-driven decisions.
f) Real-Time Data Processing: Data lakes can handle high-velocity data streams, allowing organizations to process and analyze data in near real-time, supporting real-time decision-making and operational intelligence.
g) Data Monetization: By consolidating and analyzing data from various sources, organizations can identify new revenue streams and business opportunities.
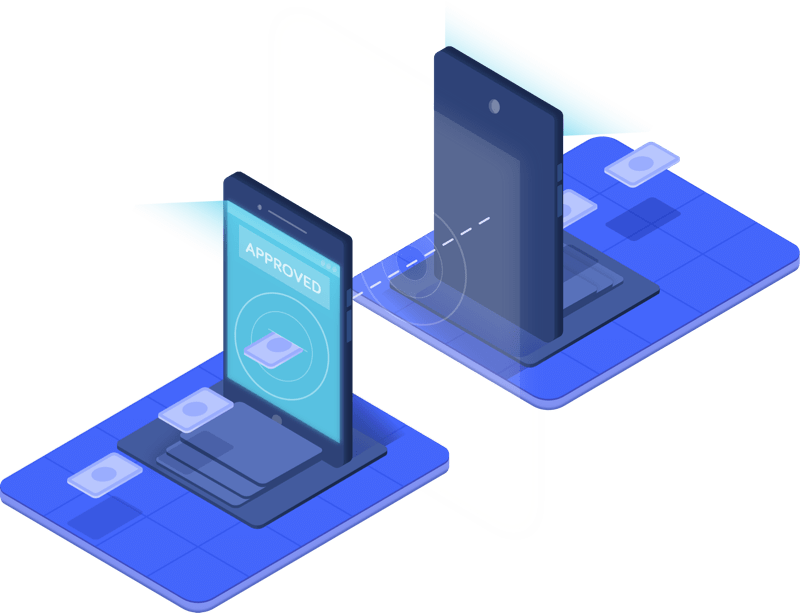
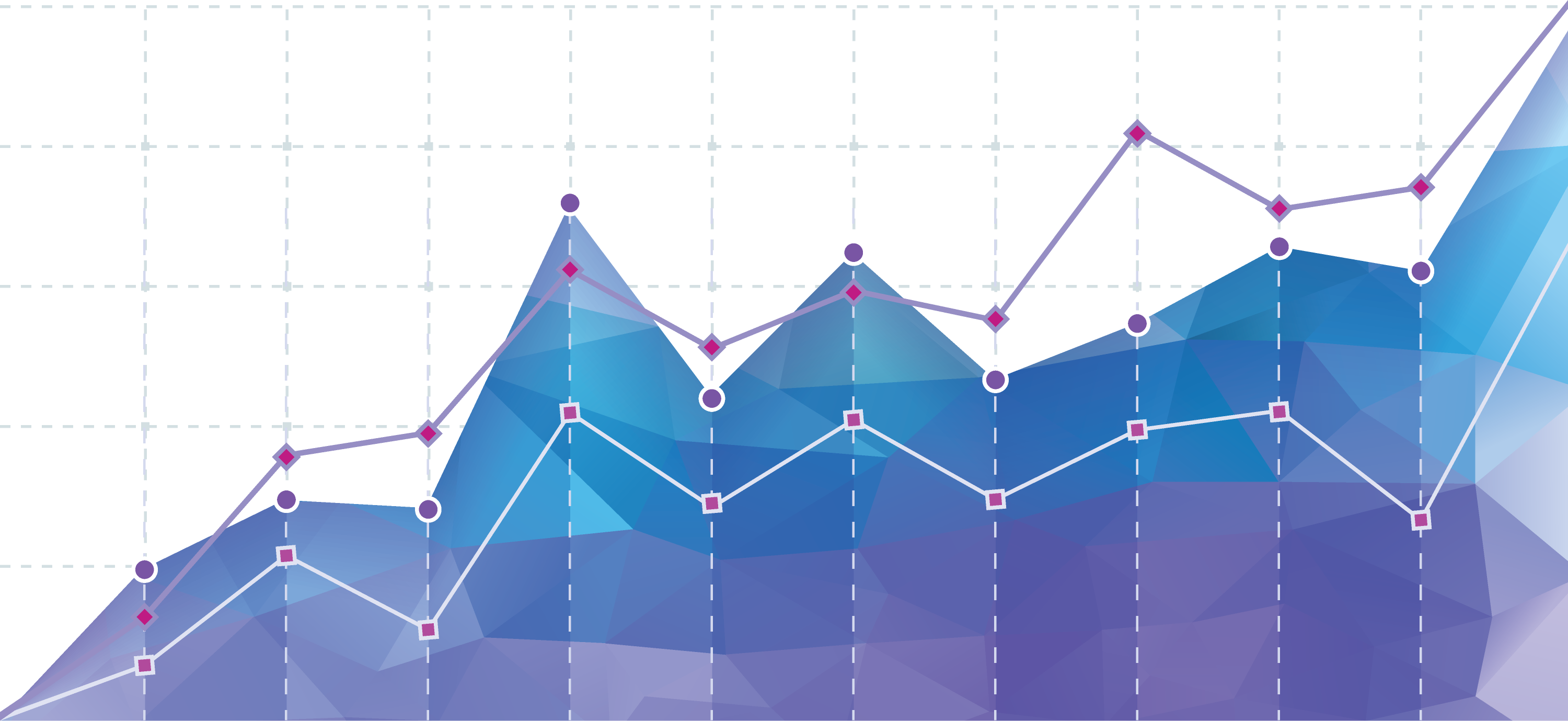
Data lakes possess several key characteristics that distinguish them from traditional data storage and management approaches:
a) Scalability: Data lakes are highly scalable, allowing organizations to seamlessly handle massive volumes of data. They can easily accommodate data growth by leveraging cloud-based storage and computing resources.
b) Flexibility: Data lakes offer flexibility in terms of data ingestion, supporting various data types and formats, including structured, semi-structured, and unstructured data. This versatility allows businesses to explore and analyze diverse data sources without requiring upfront data transformations.
c) Centralization: Data lakes serve as a central repository for all types of data within an organization, eliminating data silos and enabling cross-functional analysis.
d) Agility: Data lakes enable agile data exploration and analysis by providing users with the ability to quickly access and process data without the constraints of predefined schemas. This promotes faster insights and decision-making.
e) Cost-effectiveness: Data lakes leverage cloud-based infrastructure, allowing organizations to optimize costs by only paying for the storage and processing resources they use.
f) Advanced Analytics Capabilities: Data lakes provide a foundation for advanced analytics techniques, including machine learning, predictive analytics, and data mining, by offering a rich and diverse data set for analysis.
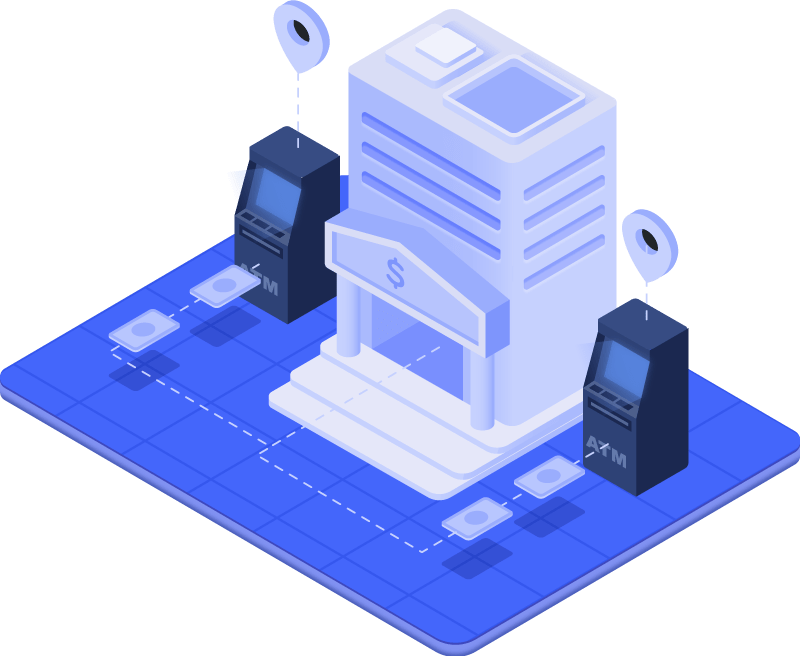
Highering AI
Success in creating AI would be the biggest event in human history. Unfortunately, it might also be the last, unless we learn how to avoid the risks.
Stephen Hawking
© 2022 Highering LLC
MEET HIGHERING AI
NEED HELP?